Lessons Learned from a Project Management Ninja: Generative AI vs Traditional IT Projects
- Datnexa HQ
- Jun 14, 2024
- 2 min read
Introduction
The evolution of generative AI marks a significant shift in technology, distinguishing it starkly from traditional IT projects. Unlike conventional computer science, which is science-led and hypothesis-driven, generative AI is engineer-led, relying on probability and prediction.
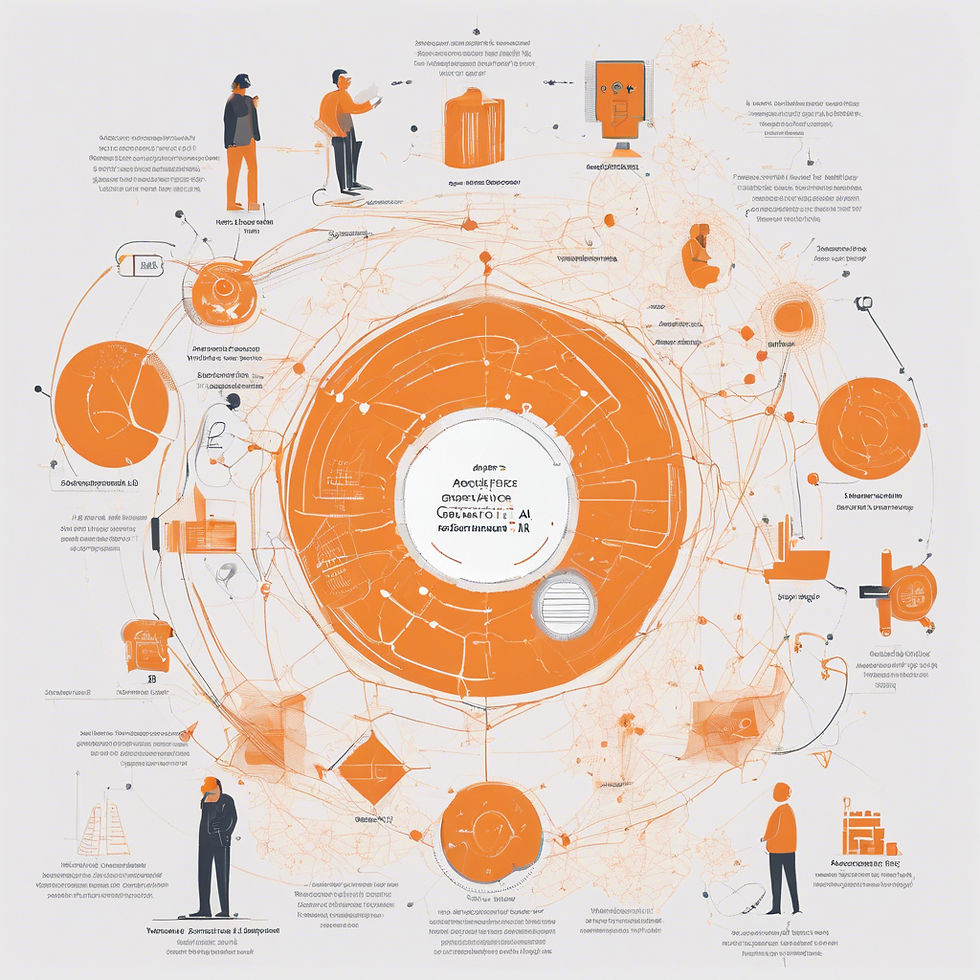
Science-led vs Engineer-led
In traditional computer science, projects are guided by established principles and hypotheses. This predictable nature allows for straightforward progress, where ticking a box or writing code yields anticipated changes. Complexity arises from the sheer volume of tasks rather than from the nature of the tasks themselves.
Conversely, AI engineering operates on probability. Generative AI functions like an advanced autocorrect, predicting the next word or the best answer based on vast datasets. This prediction-based approach requires different project management techniques, focusing on strategic goals and iterative refinement.
Project Management Implications
The shift from science-led to engineer-led projects necessitates a rethinking of traditional IT project management. Key differences include:
1. Quality Assurance and UAT - Generative AI projects demand a robust UAT phase. Implementing AI quickly provides initial solutions, but refining these solutions to perfection takes time.
2. Iterative Process - Continuous improvement through tweaking prompts and content is essential. This iterative process helps in honing the AI to meet the desired outcomes.
3. Strategic Goals and Problem Understanding - A clear understanding of the problem and strategic goals is crucial. This ensures that the AI's outputs align with the intended objectives.
Tools and Practices
Using tools like Quick Action from Agilisys can streamline the implementation of generative AI. This versatile tool functions as a Swiss Army knife for AI, handling various tasks efficiently. However, the focus should remain on:
1. Quality Assurance - Ensuring the outputs are accurate and meet the project goals.
2. Continuous Improvement - Regularly tweaking and refining the AI's performance.
3. Staff Adoption - Ensuring that the team is well-versed in using and benefiting from AI technologies.
Broader Implications
The integration of generative AI will inevitably alter the landscape of IT project management and the digital workforce. This engineer-led technology will drive social change, affecting not only IT professionals but also the wider workforce. Engaging with and refining AI technologies will be crucial for leveraging their full potential and ensuring they work for us.
Generative AI represents a paradigm shift from traditional IT project management. Embracing this change involves more than just adopting new tools; it requires a strategic, iterative approach to ensure that AI technologies deliver their full potential. By focusing on quality assurance, continuous improvement, and staff adoption, organisations can successfully integrate generative AI, driving efficiency and innovation.