In a recent session focusing on AI and data governance, several topics sparked significant discussion, providing insights into the most pressing issues facing professionals in the field. This article delves into the hottest topics discussed during the session to uncover key themes and perspectives.
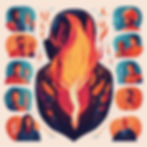
1. Managing Expectations and Stakeholder Engagement
One of the most discussed topics was managing expectations, particularly among senior managers. As AI technology continues to evolve, stakeholders often have high expectations for its capabilities, which may not always align with reality. Participants emphasized the importance of open communication and involving stakeholders early in the process to manage expectations effectively.
Another related theme was stakeholder engagement, especially with the public and internal teams. The session highlighted the need for collaboration, clear communication, and public trust-building. Engaging stakeholders through consultations and feedback mechanisms ensures that AI projects meet the needs of all involved.
2. Data Quality and Consistency
Data quality and consistency emerged as critical factors in successful AI projects. Poor-quality data can lead to biased outcomes, undermining the reliability of AI technology. The session noted that up to 80% of time in AI projects is spent on data quality, indicating its significance in achieving consistent results.
Participants discussed the importance of data validation throughout the AI lifecycle. This includes ensuring replicability and consistency in AI outputs and detecting model drift. Effective data governance practices are essential for maintaining high data quality and preventing unintended consequences.
3. The Role of Data Protection Impact Assessments (DPIAs)
The session extensively discussed DPIAs, highlighting their role in identifying and mitigating data risks. Given AI's evolving nature, DPIAs should be living documents that adapt as projects progress. Participants emphasized the need to revisit DPIAs regularly and ensure they are aligned with IT compliance.
The session also explored the relationship between DPIAs and other data governance tools, such as Equalities Impact Assessments (EAIAs). These tools play a crucial role in identifying potential biases and ensuring AI projects meet ethical standards.
4. Human Oversight and Ethical Considerations
Human oversight in AI decision-making was a significant topic of discussion. The session underscored the importance of quality assurance and human involvement to ensure AI outputs align with ethical and legal standards. This includes integrating human judgment into AI workflows to prevent unintended consequences.
Ethical considerations, such as transparency and explainability, were also prominent themes. Participants discussed the need for clear explanations of AI decisions and accountability mechanisms when things go wrong. Ensuring AI processes are transparent and that individuals understand how AI technology works helps build trust and mitigate risks.
5. Public Engagement and Building Trust
Public engagement was another hot topic, with participants highlighting the need to involve the public in AI projects. Building public trust requires transparency and clear communication about AI's purpose and expected outcomes. Regular updates and feedback mechanisms, such as online surveys or focus groups, can help engage the public and ensure their concerns are addressed.
Conclusion
The session on AI and data governance provided valuable insights into the most discussed topics in the field. Managing expectations, data quality, human oversight, and public engagement were among the hottest themes. By addressing these key areas, organizations can ensure successful AI implementations that align with ethical standards and deliver positive outcomes.